Frequency-specific age-related changes in the amplitude of spontaneous fluctuations in autism
Introduction
Autism spectrum disorder (ASD) is an early-onset neurodevelopmental disorder characterized by core deficits in social interaction and communication, along with restricted and repetitive behaviors and interests (1). To date, the etiology and pathology underlying ASD are unclear. Several efforts have been made to elucidate the neurobiology of ASD using resting-state functional magnetic resonance imaging (rs-fMRI), and most rs-fMRI studies have focused on exploring intrinsic functional connectivity (iFC) by examining the synchronicity in spontaneous activity among brain regions. The iFC studies have improved the understanding of the neurobiology of ASD. Nonetheless, some limitations in these iFC studies need to be addressed.
Typically, rs-iFC studies mainly detect synchronous abnormalities between two brain regions in ASD patients. For the abnormal iFC detected between the two regions, the region which has abnormal function and leads to asynchrony between the two regions is yet to be identified. In addition, defining regions of interest (ROIs) is also a challenging task in iFC studies. Smith et al. (2) found that results derived from an inaccurate ROI definition may lead to iFC analyses with extremely low sensitivity.
Compared to the iFC method, the amplitude of low-frequency fluctuation (ALFF) has been used as a promising metric to uncover the regional brain function changes in ASD and other psychiatric disorders. This is a regional measure examining the magnitude of low-frequency oscillations in brain activity within a specific frequency range (for example, 0.01–0.1 Hz) (3) and has been reported to have temporal stability, test-retest reliability, and replicability (4,5).
Several studies have reported abnormal ALFF in individuals with ASD in the past decade. For example, enhanced global mean ALFF values (6) and increased fractional ALFF (fALFF) in the right dorsal superior frontal cortex (7) were reported in the ASD group relative to the typically developing (TD) group. In addition, reduced ALFF values were reported in ASD studies. Guo et al. demonstrated decreased ALFF in the right precuneus and left middle occipital gyrus (8) in ASD. Jung et al. reported reduced fALFF in the right cuneus and lateral occipital cortex (9) in the ASD group relative to the TD group.
Previous studies have shown that ALFF is an effective method to detect regional functional abnormalities in ASD; however, inconsistent findings are also observed in these studies. For example, different impaired brain regions were reported in ASD individuals using ALFF. In addition, both increased and decreased ALFF were detected in ASD. One possible factor contributing to these mixed results is that ALFF abnormalities are frequency-dependent. Another possibility is that developmental changes were largely ignored in previous ASD studies using ALFF.
To date, although some previous ASD studies examined the disruption of spontaneous low-frequency oscillations (LFO), the majority focused on the conventional low-frequency range (0.01–0.1 Hz). However, some studies found that oscillations within specific frequency bands are linked to various neural processes and physiological functions and have distinct spatial profiles (10). The LFO is classified into four distinct frequency bands [slow-5 (0.01–0.027 Hz), slow-4 (0.027–0.073 Hz), slow-3 (0.073–0.198 Hz), and slow-2 (0.198–0.25 Hz)], and Zuo et al. found that LFO amplitudes in the slow-4 band were robust in the basal ganglia, with amplitudes in the slow-5 band were dominant within medial prefrontal cortices (11). In the field of ASD, some studies have explored the rs-iFC across these low-frequency bands. For example, Duan et al. conducted cortical network iFC analyses in adolescents with ASD at slow-5, slow-4, and slow-3 frequency bands (12). The study observed decreased connectivity between the dorsal attention network (DAN) and ventral attention network (VAN) in the lower frequency bands (slow-5 and slow-4) in addition to decreased connectivity between the limbic network (LN) and frontal-parietal network (FPN) in the higher frequency band (slow-3). Nonetheless, none of the studies have examined whether atypical ALFF values are related to specific frequency bands in the ASD population.
Developmental changes in the brains of ASD patients have received increasing attention. Over the past decade, MRI studies have provided evidence of age-related variations in brain anatomy, function, and connectivity, which improves the understanding of the neurobiology of ASD. Only one has examined developmental changes in individuals with ASD using ALFF among the studies using regional measures. Guo et al. explored the maturation trajectory in ASD participants aged 7–39-year-old and found lower ALFF in children and higher ALFF in adolescents and adults with ASD in the medial prefrontal cortex (mPFC) than in TD individuals (8). Notably, the multiple comparison correction strategies used in this study may not be stringent (13). Moreover, the frequency-specific developmental changes of LFO in ASD were not explored.
In the current study, we applied ALFF to explore the developmental changes in the amplitudes of LFO in individuals with autism aged 6–30-year-old. We also examined whether the changes were frequency-dependent [across slow-5 (0.01–0.027 Hz), slow-4 (0.027–0.073 Hz), and slow-3 (0.073–0.1 Hz)] and predicted that abnormalities in LFO in autism were related to age and maybe frequency-dependent. The present study was a preliminary exploration to reveal that the influence of age and frequency bands on regional brain activities contributes to the psychopathology mechanism underlying autism. We present the following article in accordance with the MDAR checklist (available at https://tp.amegroups.com/article/view/10.21037/tp-21-412/rc).
Methods
Ethical statements
The study was conducted in accordance with the Declaration of Helsinki (as revised in 2013). Our experimental protocol was approved by the ethics committee of Peking University Sixth Hospital (No. 15010000001498283). All procedures were carried out in accordance with the approved guidelines and regulations. For participants aged <8 years or with intelligence <8-year-old, written informed consent was obtained from the parents/legal guardians, and for participants >8-year-old or with intelligence that of >8-year-old, written informed consent was obtained from both participants and their parents/legal guardians.
Participants
For the current study, participants with autism were recruited from the outpatient clinic at the Institute of Mental Health, Peking University, China, and TD participants were recruited through advertising and word of mouth. A total of 111 autistic and 59 TD right-handed subjects, aged 6–30-year-old were enrolled in this study. All subjects had a full-scale intelligence quotient (FIQ) >70, which was estimated by either the Chinese-Wechsler Intelligence Scale for Children (C-WISC) (6.0–16.9-year-old) (14) or the Wechsler Adult Intelligence Scale-Revised in China (WAIS-RC) (17.0–30.0-year-old) (15). All participants with autism were diagnosed according to the autistic disorder criteria of Diagnostic and Statistical Manual-Ⅳ (DSM-Ⅳ) by two experienced child and adolescent psychiatrists (16). Participants were excluded if they had a history of other psychiatric disorders, major physical or neurological illness, a history of head injury or alcohol or drug abuse, previous or current psychotropic medication, or metalwork (for example, metal braces and cardiac pacemakers). Subjects with a mean framewise displacement (FD) (17) >2 standard deviations above the mean FD of the whole sample (0.713 mm) were excluded. The final cohort included 65 individuals in the autism group and 55 in the TD group. No differences were detected in age (t=−1.314, P=0.191) or gender (χ2=2.760, P=0.097) between the two groups (Table 1).
Table 1
Autism (n=65) | TD (n=55) | t/χ2/z | P value | |
---|---|---|---|---|
Sex (N) | 2.76 | 0.097 | ||
Male | 56 | 40 | ||
Female | 9 | 15 | ||
Handedness (right/left) | 65/0 | 55/0 | ||
Agea | 13.28±4.99 | 14.48±5.95 | −1.31 | 0.191 |
Full-scale IQa | 103±17 | 115±11 | −4.52 | <0.0001 |
Verbal IQa | 108±19 | 116±13 | −2.72 | 0.006 |
Performance IQa | 96±16 | 111±12 | −5.52 | <0.0001 |
Head Motion (mean FD)c | 0.116 | 0.059 | −3.30 | 0.001d |
Medications (No/Yes) | 65/0 | 55/0 |
a, mean ± SD; b, n=44; c, median; d, asymptotic significance was displayed using a nonparametric test. IQ, intelligence quotient; mean FD, mean framewise displacement; TD, typical development; SD, standard deviation.
Data acquisition
MRI data were collected on a GE Discovery MR750 3T scanner with an 8-channel head coil at the Peking University Third Hospital. Spontaneous brain activity was measured with an 8-min rs-fMRI scan consisting of 240 contiguous whole-brain functional volumes using a multi-echo echo-planar imaging (EPI) sequence [repetition time (TR) =2.0 s; echo time (TE) =20 ms; flip angle =90°; 41 slices; matrix =64×64; voxel size = 3.75 mm × 3.75 mm × 3.30 mm].
fMRI preprocessing
Data preprocessing was carried out using the Data Processing Assistant for Resting-State fMRI (DPARSF) (18), which is based on the toolbox of Data Processing and Analysis for Brain Imaging (DPABI) (19) and Statistical Parametric Mapping (SPM). The processing steps included discarding the first 10 volumes, slice timing correction, spatial head motion realignment using a six-parameter rigid-body linear transformation, normalization to the MNI space, nuisance covariate regression (Friston 24-parameter motion model (20), white matter (WM), and cerebrospinal fluid (CSF) signal, and the linear trend removal.
Data analysis
ALFF calculation
Voxel-wise whole-brain regional activities were mapped for each participant using ALFF (3). This method examines the mean of amplitudes within a specific frequency range (0.01–0.1 Hz) of the spectrum that transformed the voxel time course using fast Fourier transformation. Next, we detected the effect of the widely used low-frequency band (0.01–0.1 Hz) ALFF that was further categorized into three frequency bands to detect frequency-specific effects: slow-5 (0.01–0.027 Hz), slow-4 (0.027–0.073 Hz), and slow-3 (0.073–0.1 Hz) (11). For further analyses, ALFF maps were normalized using z-standardization (subtracting the mean value for the entire brain from each voxel and dividing this result by the corresponding SD) and smoothed (4 mm FWHM).
Group-level statistics
Statistical analysis of the clinical data was performed using IBM SPSS Statistics 23. Based on DPABI, we used the general linear model (GLM) to examine ALFF and specific-band ALFF differences between autism and TD individuals with the diagnostic group, age, diagnostic group-by-age, sex, FIQ, and mean FD as regressors. According to Eklund et al. (13), correcting multiple comparisons with a loose threshold leads to an unacceptable familywise error rate. Chen et al. suggested that a permutation test with threshold-free cluster enhancement (TFCE), a strict method, can maintain the familywise error rate under 5% and achieve the best reproducibility (5). Herein, we utilized a permutation test (5,000 permutations) (13,21) with TFCE (two-tailed, threshold of P values =0.05) (22) to correct the results. Global signal regression (GSR) is still controversial in the rs-fMRI field, and analyses with and without GSR are considered complementary (23). To confirm that none of the results were altered by GSR, we repeated the group-level analysis with the ALFF images preprocessed with GSR. The statistical data were similar to their counterparts, preprocessed without GSR. The relevant results are displayed in Tables S1,S2 and Figure S1.
In order to investigate the shape of the developmental trajectories of the autism and TD groups, we calculated the mean ALFF values in each cluster, which displayed significant diagnostic group-by-age interactions. Then, a linear regression model in SPSS was used to explore the correlation between ALFF and age from which the irrelevant covariates (sex, FIQ, and mean FD) were regressed out. In addition, for the clusters exhibiting significant group-by-age interactions, we divided the subjects into three cohorts according to age (childhood, 6.0–11.9 years, n=49; adolescence, 12.0–17.9 years, n=45; adulthood, 18.0–30.0 years, n=26) to elucidate the differences between diagnostic groups (Table S3). One-way analysis of variance (ANOVA) and post-hoc analysis was used to explore the differences among the three stages from childhood to early adulthood in the autism and TD groups.
The correlation between regional activity and symptom severity assessed by the parent-report questionnaires was analyzed, but no significant results were found (for details, see Appendix 1).
Significant differences of all statistical analyses were considered at P<0.05 (two-sided).
Results
Main effect and diagnostic group-by-age interaction
Two-sample t-test analysis showed that the diagnostic group did not influence ALFF (0.01–0.1 Hz) (P>0.05). However, significant diagnostic group-by-age interactions were found in two clusters: Caudate and Putamen (PT with TFCE, P<0.05, corrected) (Table 2, Figure 1).
Table 2
Cluster size (Voxels) | Peak MNI | Max intensity (z) | Region(s)a,c | ||||
---|---|---|---|---|---|---|---|
x | y | z | Regionb | ||||
Cluster 1 | 36 | 9 | 18 | −6 | Caudate_R | −4.180 | Right Putamen, right Caudate nucleus, right Frontal Lobe, right Lentiform Nucleus |
Cluster 2 | 50 | 27 | −12 | −9 | – | −4.421 | Right Lentiform Nucleus, right Putamen, right Pallidum, right Temporal Lobe, right Lateral Globus Pallidus, right Medial Globus Pallidus |
a, Cluster size ≥5 voxels; b, Brain regions as defined with Anatomical Automatic Labeling (AAL); c, Brain regions as defined with Harvard-Oxford Subcortical Atlas and/or Harvard-Oxford Cortical Structural Atlas. ALFF, amplitude of low-frequency fluctuations; Cluster 1: Caudate cluster; Cluster 2: Putamen cluster.
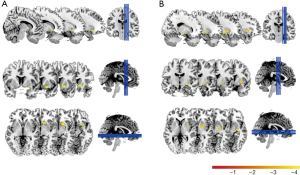
Next, we examined if our results were frequency-dependent and found a significant diagnostic group-by-age interaction in a cluster in the frequency band slow-4 (0.027–0.073 Hz) (Figure 2); it was nearly in the same position with the Caudate and Putamen clusters. No significant cluster was found in the other two bands.
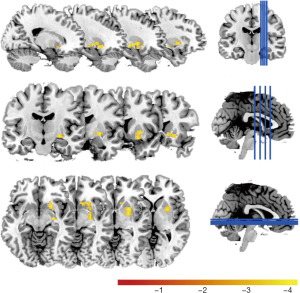
Shape of developmental trajectories
For the two clusters showing diagnostic group-by-age interaction effects in ALFF, namely, the Caudate and the Putamen clusters, the shape of the developmental trajectories was investigated by comparing linear fits in autism and TD groups independently. For the autism group, the linear fits were significant in both the Caudate cluster (t=−5.605, P<0.001, R2=0.333) (Figure 3A) and the Putamen cluster (t=−3.433, P=0.001, R2=0.158) (Figure 3B). However, the linear models were not significant in either cluster in the TD group (Caudate cluster: t=0.393, P=0.696; Putamen cluster: t=1.544, P=0.128).
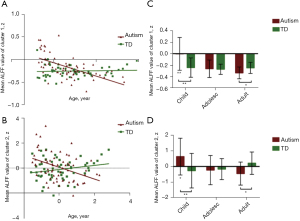
Group differences in each age cohort
The group comparison of ALFF values result in each age cohort for both clusters showed hyperactivity in the autism group in childhood compared to the TD group (Caudate cluster: t=3.626, P=0.001; Putamen cluster: t=2.839, P=0.007) and hypoactivity in adulthood relative to the TD group (Caudate cluster: t=−2.198, P=0.038; Putamen cluster: t=−2.314, P=0.030), while no significant differences were detected during adolescence (all P>0.05) (Figure 3C,3D).
Age stratification differences in the autism and TD groups
The comparison of the mean ALFF z-values in different age cohorts in each group revealed significant differences in both the Caudate and Putamen clusters (Caudate cluster: F=13.992, P<0.001; Putamen cluster: F=6.776, P=0.002) in the autism group, while TD individuals did not show any significant effects (both P>0.05). Specifically, the values were significantly higher for autistic children than autistic adolescents and adults, whereas no significant differences were detected between autistic adolescents and autistic adults in either the Caudate cluster (childhood > adolescence, Bonferroni-corrected, P<0.001; childhood > adulthood, Bonferroni-corrected, P<0.001; adolescence > adulthood, Bonferroni-corrected, P=1.000) or Putamen cluster (childhood > adolescence, Bonferroni-corrected, P=0.008; childhood > adulthood, Bonferroni-corrected, P=0.012; adolescence > adulthood, Bonferroni-corrected, P=1.000) (Figure 3C,3D).
Discussion
In the current study, we examined the developmental trajectories of ALFF in individuals diagnosed with autism compared to those in TD individuals aged 6–30-year-old. The current results showed significant group-by-age interaction effects in the dorsal striatum, with remarkably higher ALFF in autistic children and lower ALFF in autistic adults than TD peers. We also examined if those age-related changes were frequency-dependent [across slow-5 (0.01–0.027 Hz), slow-4 (0.027–0.073 Hz) and slow-3 (0.073–0.1 Hz)], and found abnormal ALFF values specific to the slow-4 frequency band. Therefore, our results emphasized inspecting both age-related and frequency-specific changes of spontaneous regional activities in autism.
The current findings were consistent with the results of Supekar et al., wherein high local activity signals were observed in the subcortical regions using ALFF in children with autism. In addition, Itahashi et al. also reported reduced regional activity in the right middle occipital gyrus, lingual gyrus, and fusiform gyri in adults with autism (24). However, Guo et al. observed opposite results similar to our study, showing increasing ALFF values with age in the mPFC in ASD and reduced ALFF values in children with ASD relative TD (8). These inconsistencies could be attributed to several differences in methodology (5) and ethnicity (24). However, additional studies probing age-related regional spontaneous activity changes in ASD are required.
As described above, the current results showed significant age-by-group interaction in the dorsal striatum with increased ALFF in children and decreased in adults with autism compared to TD. An individual with autism exhibits altered regional activities in the striatum, which could be ascribed to structural abnormalities. One review suggested a link between structural and functional abnormalities in the brain, indicating that developmentally abnormal brain regions might be unable to function effectively (25). Previous studies have demonstrated that the striatum, especially the caudate nucleus, showed atypical age-related changes in the volume in ASD (26-28). Therefore, the developmental structural abnormalities in the striatum might underlay the age-related alterations of ALFF in autism in the present study.
In the current study, hyperspontaneous activity within the caudate and putamen in children with autism was consistent with the gate deficit theory (29-31). The dysfunction of the striatum in the gating circuit might fail to filter out the irrelevant sensory stimuli, resulting in overwhelming sensory information reaching the cortex in autism, which could be associated with repetitive behaviors and sensory deficits. To counterbalance this situation, regional spontaneous overactivity or overconnectivity within the gating circuit such as striatum could suppress the overwhelming information (29-31). Therefore, hyperactivity in the dorsal striatum region could form one of the bases of compensatory circuits in ASD children. In addition, hyperactivity may synchronize with the overconnectivity in children with ASD, supporting the overconnectivity model in autistic children, as reported previously (6).
However, this synchrony could not be explained in adolescence or adulthood autism, which could occur due to the developmental complexity in these stages. Specifically, the second growth spurt in adolescence leads to dramatic changes in behavior and neural organization, rendering it a “second hit” on an already weak system in individuals with ASD (32). Due to this “second hit,” the compensatory mechanisms cannot catch up with the rapid speed of development, thereby leading to a marked decline of the spontaneous activity during adolescence in the dorsal striatum, as seen in our study. As a result, the overactivity of ALFF in children was diminished in adolescents and even reversed as underactivity in adults with ASD. The pattern that differential developmental trajectories diverged in adulthood observed in the ASD and TD groups in the current study was referred to as multifinality (33). In a previous study, the abnormal pattern revealed developmental derangement or delayed development in ASD individuals (34). The deviation in the shape of the maturation trajectories found in our study suggests profound developmental disruptions in the dorsal striatum that began in the early years and persisted into adulthood in ASD. The existence of this incongruous maturational pattern highlights a complex profile of age-related alterations in spontaneous regional activity in ASD.
In the current study, we further examined ALFF values that showed significant age-by-group interactions across slow-5, slow-4, and slow-3 bands. The findings demonstrated significant age-by-group interaction effects only in the slow-4 band. Therefore, our study showed that abnormal age-related regional spontaneous activities in autism were frequency-dependent. These findings were consistent with accumulating evidence that the abnormal regional brain activity was frequency-sensitive in some neuropsychiatric disorders. For example, patients with schizophrenia showed widespread abnormal ALFF amplitudes in the slow-4 frequency band (35). Regarding attention-deficit/hyperactivity disorder (ADHD), Di Martino et al. found that fluctuations in the slow-4 frequency band provided better diagnostic information than global band deviations in children with ADHD (36). Our study showed, for the first time, that age-related regional spontaneous activity abnormalities in individuals with autism were specific to the slow-4 frequency band and were located in the striatum. As shown in another study, the functional connections in the slow-4 frequency band were most sensitive for discriminating ASD individuals from TD (37); these findings indicate the potential of slow-4 frequency as a stable autism biomarker, although additional investigation is essential.
Nevertheless, the present findings should be interpreted with some limitations. First, the current research used a cross-sectional design; a longitudinal study design is the gold standard to elucidate the brain variability with age. Second, children <6-year-old with ASD should be under intensive focus because their largest developmental alterations may occur earlier. Third, since the research reliability is affected by the statistical power of the sample size, it is necessary to enlarge the sample in our study, especially the adult cohort.
Conclusions
In conclusion, from a developmental perspective, the current study found altered age-related ALFF values in the dorsal striatum in autism. Moreover, the abnormal ALFF values were specific to the slow-4 frequency band. Our findings highlighted the potentially significant role of the striatum and the slow-4 frequency band in the pathology of autism; however, these findings need to be substantiated with additional studies.
Acknowledgments
Funding: This work was supported by National Key R&D Program of China (No. 2017YFC1309900); Beijing Municipal Science and Technology Commission (No. Z181100001518005, 7164314); the National Natural Science Foundation of China (No. 81271508); the Key Research Program of the Chinese Academy of Sciences (No. ZDBS-SSW-JSC006); and Beijing Nova Program of Science and Technology (No. Z191100001119104).
Footnote
Reporting Checklist: The authors have completed the MDAR checklist. Available at https://tp.amegroups.com/article/view/10.21037/tp-21-412/rc
Data Sharing Statement: Available at https://tp.amegroups.com/article/view/10.21037/tp-21-412/dss
Conflicts of Interest: All authors have completed the ICMJE uniform disclosure form (available at https://tp.amegroups.com/article/view/10.21037/tp-21-412/coif). The authors have no conflicts of interest to declare.
Ethical Statement: The authors are accountable for all aspects of the work in ensuring that questions related to the accuracy or integrity of any part of the work are appropriately investigated and resolved. The study was conducted in accordance with the Declaration of Helsinki (as revised in 2013). The study was approved by the Ethical Committee of Peking University 6th Hospital (No. 15010000001498283). For participants aged <8 years or with intelligence <8-year-old, written informed consent was obtained from the parents/legal guardians, and for participants >8-year-old or with intelligence that of >8-year-old, written informed consent was obtained from both participants and their parents/legal guardians.
Open Access Statement: This is an Open Access article distributed in accordance with the Creative Commons Attribution-NonCommercial-NoDerivs 4.0 International License (CC BY-NC-ND 4.0), which permits the non-commercial replication and distribution of the article with the strict proviso that no changes or edits are made and the original work is properly cited (including links to both the formal publication through the relevant DOI and the license). See: https://creativecommons.org/licenses/by-nc-nd/4.0/.
References
- American Psychiatric Association. Diagnostic and statistical manual of mental disorders, 5th ed: Washington, DC: American Psychiatric Association 2013.
- Smith SM, Miller KL, Salimi-Khorshidi G, et al. Network modelling methods for FMRI. Neuroimage 2011;54:875-91. [Crossref] [PubMed]
- Zang YF, He Y, Zhu CZ, et al. Altered baseline brain activity in children with ADHD revealed by resting-state functional MRI. Brain Dev 2007;29:83-91. [Crossref] [PubMed]
- Zuo XN, Kelly C, Di Martino A, et al. Growing together and growing apart: regional and sex differences in the lifespan developmental trajectories of functional homotopy. J Neurosci 2010;30:15034-43. [Crossref] [PubMed]
- Chen X, Lu B, Yan CG. Reproducibility of R-fMRI metrics on the impact of different strategies for multiple comparison correction and sample sizes. Hum Brain Mapp 2018;39:300-18. [Crossref] [PubMed]
- Supekar K, Uddin LQ, Khouzam A, et al. Brain hyperconnectivity in children with autism and its links to social deficits. Cell Rep 2013;5:738-47. [Crossref] [PubMed]
- Di Martino A, Yan CG, Li Q, et al. The autism brain imaging data exchange: towards a large-scale evaluation of the intrinsic brain architecture in autism. Mol Psychiatry 2014;19:659-67. [Crossref] [PubMed]
- Guo X, Chen H, Long Z, et al. Atypical developmental trajectory of local spontaneous brain activity in autism spectrum disorder. Sci Rep 2017;7:39822. [Crossref] [PubMed]
- Jung M, Tu Y, Lang CA, et al. Decreased structural connectivity and resting-state brain activity in the lateral occipital cortex is associated with social communication deficits in boys with autism spectrum disorder. Neuroimage 2019;190:205-12. [Crossref] [PubMed]
- Buzsáki G, Draguhn A. Neuronal oscillations in cortical networks. Science 2004;304:1926-9. [Crossref] [PubMed]
- Zuo XN, Di Martino A, Kelly C, et al. The oscillating brain: complex and reliable. Neuroimage 2010;49:1432-45. [Crossref] [PubMed]
- Duan X, Chen H, He C, et al. Resting-state functional under-connectivity within and between large-scale cortical networks across three low-frequency bands in adolescents with autism. Prog Neuropsychopharmacol Biol Psychiatry 2017;79:434-41. [Crossref] [PubMed]
- Eklund A, Nichols TE, Knutsson H. Cluster failure: Why fMRI inferences for spatial extent have inflated false-positive rates. Proc Natl Acad Sci U S A 2016;113:E4929. [Crossref] [PubMed]
- Gong YX. Manual of Wechsler Adult Intelligence Scale - Revised in China. Changsha: Hunan Atlas Press 1992.
- Gong YX, Cai TS. The Wechsler Intelligence Scale for Children Revised in China (C-WISC). Chinese Journal of Clinical Psychology 1993;2:1-7.
- American Psychiatric Association. Diagnostic and Statistical Manual of Mental Disorders, 4th edn: American Psychiatry Association, Washington, DC 1994.
- Jenkinson M, Bannister P, Brady M, et al. Improved optimization for the robust and accurate linear registration and motion correction of brain images. Neuroimage 2002;17:825-41. [Crossref] [PubMed]
- Chao-Gan Y, Yu-Feng Z. DPARSF: A MATLAB Toolbox for "Pipeline" Data Analysis of Resting-State fMRI. Front Syst Neurosci 2010;4:13. [PubMed]
- Yan CG, Wang XD, Zuo XN, et al. DPABI: Data Processing & Analysis for (Resting-State) Brain Imaging. Neuroinformatics 2016;14:339-51. [Crossref] [PubMed]
- Friston KJ, Williams S, Howard R, et al. Movement-related effects in fMRI time-series. Magn Reson Med 1996;35:346-55. [Crossref] [PubMed]
- Winkler AM, Ridgway GR, Douaud G, et al. Faster permutation inference in brain imaging. Neuroimage 2016;141:502-16. [Crossref] [PubMed]
- Smith SM, Fox PT, Miller KL, et al. Correspondence of the brain's functional architecture during activation and rest. Proc Natl Acad Sci U S A 2009;106:13040-5. [Crossref] [PubMed]
- Murphy K, Fox MD. Towards a consensus regarding global signal regression for resting state functional connectivity MRI. Neuroimage 2017;154:169-73. [Crossref] [PubMed]
- Itahashi T, Yamada T, Watanabe H, et al. Alterations of local spontaneous brain activity and connectivity in adults with high-functioning autism spectrum disorder. Mol Autism 2015;6:30. [Crossref] [PubMed]
- Ecker C, Bookheimer SY, Murphy DG. Neuroimaging in autism spectrum disorder: brain structure and function across the lifespan. Lancet Neurol 2015;14:1121-34. [Crossref] [PubMed]
- Langen M, Schnack HG, Nederveen H, et al. Changes in the developmental trajectories of striatum in autism. Biol Psychiatry 2009;66:327-33. [Crossref] [PubMed]
- Langen M, Bos D, Noordermeer SD, et al. Changes in the development of striatum are involved in repetitive behavior in autism. Biol Psychiatry 2014;76:405-11. [Crossref] [PubMed]
- Ma ZH, Lu B, Li X, et al. Atypicalities in the developmental trajectory of cortico-striatal functional connectivity in autism spectrum disorder. Autism 2021; [Epub ahead of print]. [Crossref] [PubMed]
- Cerliani L, Mennes M, Thomas RM, et al. Increased Functional Connectivity Between Subcortical and Cortical Resting-State Networks in Autism Spectrum Disorder. JAMA Psychiatry 2015;72:767-77. [Crossref] [PubMed]
- Perry W, Minassian A, Lopez B, et al. Sensorimotor gating deficits in adults with autism. Biol Psychiatry 2007;61:482-6. [Crossref] [PubMed]
- Uddin LQ. Salience processing and insular cortical function and dysfunction. Nat Rev Neurosci 2015;16:55-61. [Crossref] [PubMed]
- Picci G, Scherf KS. A Two-Hit Model of Autism: Adolescence as the Second Hit. Clin Psychol Sci 2015;3:349-71. [Crossref] [PubMed]
- Padmanabhan A, Lynn A, Foran W, et al. Age related changes in striatal resting state functional connectivity in autism. Front Hum Neurosci 2013;7:814. [Crossref] [PubMed]
- Di Martino A, Kelly C, Grzadzinski R, et al. Aberrant striatal functional connectivity in children with autism. Biol Psychiatry 2011;69:847-56. [Crossref] [PubMed]
- Hoptman MJ, Zuo XN, Butler PD, et al. Amplitude of low-frequency oscillations in schizophrenia: a resting state fMRI study. Schizophr Res 2010;117:13-20. [Crossref] [PubMed]
- Di Martino A, Ghaffari M, Curchack J, et al. Decomposing intra-subject variability in children with attention-deficit/hyperactivity disorder. Biol Psychiatry 2008;64:607-14. [Crossref] [PubMed]
- Chen H, Duan X, Liu F, et al. Multivariate classification of autism spectrum disorder using frequency-specific resting-state functional connectivity—A multi-center study. Prog Neuropsychopharmacol Biol Psychiatry 2016;64:1-9. [Crossref] [PubMed]