Proteomic analysis of serum proteins in children with brain death
Introduction
Since the 2010 implementation of a voluntary citizen-based organ donation program, patients suffering from brain death (BD) have emerged as the most important source of donor organs in China (1,2). BD is considered to be an important cause of pretransplantation, it causes significant changes in hemodynamics instability, and thormonal impairment and inflammation, resulting in various changes across organ systems.
Organs from donors affected by BD exhibit higher rates of graft discard than those obtained from other donor sources (3,4). Treatment and transplant procurement should therefore be conducted as soon after the detection of BD as possible (5). However, only a tiny proportion of hospitals are currently able to diagnose BD, due to the extremely strict criteria for defining BD. Further, the process for determining BD is both complex and time-consuming, which consequently impedes the timely procurement and maintenance of organs for donation. Data from the United States in 2019 indicated that BD was evident in up to 20.7% of children who died in pediatric intensive care units; most of the deceased had suffered acute hypoxic-ischemic or traumatic brain injuries and had no history of neurological dysfunction (6). Therefore, efficient identification of BD in order to make full use of donated organs constitutes an important approach to improving donation rates, reducing organ waste, and alleviating the donor shortage.
Blood samples can be readily collected and are fairly stable, making serum an ideal biofluid for biomarker detection. Cerebrospinal fluid (CSF) is closely associated with the brain, so might provide direct insight into the incidence and pathogenesis of BD; however, its collection necessitates a lumbar puncture. Therefore, analysis of routinely collected blood samples is generally considered to be more acceptable than CSF detection by patients and their families. The injury of the central nervous system could lead to the secretion of protein into the CSF, proteins that are normally present at high concentrations in the CSF can diffuse freely into the blood (7). However, these CSF-derived biomarkers are likely to remain relatively dilute, meaning they are less abundant than normal serum proteins (8).
Technological advances over the past decade have yielded novel tools capable of detecting and analyzing low-abundance circulating biomarker proteins using mass spectrometry-based approaches and proteomic analyses (9). Gao used two-dimensional difference gel electrophoresis combined with mass spectrometry to compare the serum protein profiles of children with mild AHT to those of age-matched controls, and found that SAA may be a potential biomarker to identify children with mild AHT who present for medical care without a history of trauma (10). No studies to date have explored the proteomic signatures of human BD. Therefore, the present study employed a tandem mass tag (TMT)-labeled quantitative proteomics approach to examine the serum proteome profiles of patients with BD compared to those of healthy controls (HCs), in an effort to provide a foundation for future efforts to screen for molecular biomarkers of BD. By aiding in the early identification of BD, it is hoped that our findings will have the potential to improve the success of organ donation procedures in future. We present the following article in accordance with the MDAR reporting checklist (available at https://tp.amegroups.com/article/view/10.21037/tp-21-559/rc).
Methods
Patients and samples
This study was approved by the Medical Ethics Committee of the First Affiliated Hospital of Guangxi Medical University [project identification code: 2020 (KY-E-156)] and adhered to the principles of the Declaration of Helsinki (as revised in 2013). Signed written informed consent was obtained from the parents or guardians of each participating child before study enrollment.
Both the children diagnosed with BD and age- and sex-matched HC groups were enrolled between December 2020 and March 2021. Patients in the BD group [5 males and 3 females, mean age and standard deviation (SD): 4.85±4.07 years] were designated CK1–CK8, while the HCs (3 males and 5 females, mean age and SD: 4.80±2.70 years) were designated T1–T8. All participants underwent comprehensive clinical examination, including physical and neurological examination with detailed history, neurological examination, and routine laboratory testing. BD was assessed independently by two physicians and was based on the following criteria: unexplained deep coma, absence of brainstem reflexes, apnea test, and confirmatory test with absence of cerebral blood flow, according to the Criteria and practical guidance for determination of BD in children, published in 2018 (11).
Individuals with severe cardiopulmonary insufficiency, liver or kidney dysfunction, severe malnutrition, a history of tumors or other related diseases affecting serum protein content, recent fever, inflammation, or other conditions that could potentially impact test results were excluded from the HC group.
Serum samples were collected from patients in the BD group at the time of BD diagnosis. From each patient, 5 mL of venous blood was collected using a centrifuge or vacuum tube without anticoagulants. Samples were allowed to coagulate at 4 °C for 30 minutes, and were then centrifuged at 1,600 ×g for 15–20 minutes at 4 °C. The serum was then transferred to a fresh 1.5-mL centrifuge tube and stored at −80 °C.
Protein extraction and identification
Protein extraction
The removal of >80% of albumin and immunoglobulin G (IgG) from the collected serum samples was performed using a Proteo Extract Prep Blue Albumin and IgG Depletion kit (Sigma, USA) in accordance with the directions supplied by the manufacturer. The total protein concentrations in the samples were then measured with a Bradford assay kit (Sigma, USA) following the manufacturer’s directions, using bovine serum albumin as a standard.
Trypsin digestion
Peptides (200 µg) extracted from each sample were first reduced with 5 mM dithiothreitol for 1 hour at 60 °C. They were then alkylated with 11 mM iodoacetamide for 30 minutes at room temperature while being protected from light. Trypsin was added at a mass ratio of 1:50 (trypsin:protein) overnight at 37 °C. Next, trypsin was added at a mass ratio of 1:100 (trypsin:protein), and proteolytic cleavage was allowed to continue for a further 4 hours.
TMT labeling
Following trypsin digestion, peptides were desalted using a Strata X C18 (Phenomenex) before being dissolved in 0.5 M triethylammonium bicarbonate (Sigma). A TMT kit (Thermo) was then used to label the peptides in accordance with the manufacturer’s directions. In brief, one unit of TMT reagent was thawed and reconstituted in acetonitrile. The peptide mixtures were then incubated for 1 hour at room temperature, after which they were pooled, desalted, and dried via vacuum centrifugation.
High-performance liquid chromatography fractionation and liquid chromatography with tandem mass spectrometry analysis
Trypsinized peptides were fractionated by high-pH reverse-phase high-performance liquid chromatography using a Dionex Ultimate 3000 RSLCnano instrument (Thermo Fischer Scientific). In brief, peptides were initially separated using a 5–95% acetonitrile (pH 10.0) gradient over a 90-minute period, after which they were combined into 10 fractions using vacuum centrifugation.
Samples were loaded onto a Gemini-NX pre-column (1.5 cm ×200 µm, 3 µm, C18, Phenomenex, USA). Peptide separation was performed using an Acclaim PepMap RSLC column (1.5 cm ×75 µm, 2 µm, C18, Dionex, USA) with buffer A (100% ultrapure water and 0.1% formic acid) and buffer B (80% acetonitrile and 0.1% formic acid) at a 600 nL/min flow rate. The following multi-step linear buffer B gradient protocol was used: 5% buffer B for 5 minutes, linear gradient to 10% buffer B across 5 min, followed by 40% B for 50min, step increase to 95% buffer B, 95% buffer B for 5 min, linear decrease to 5% buffer B across 10 min. Peaks were detected at 214 nm. Peptides were then assessed by tandem mass spectrometry with a Q Exactive™ Plus (Thermo Fisher Scientific) at a full-scan setting of m/z from 350 to 1,600 and a resolution of 60,000 for Orbitrap. A normalized collision energy setting of 35 was selected for intact fraction detection.
Peptide identification and quantification
Liquid chromatography with tandem mass spectrometry (LC-MS/MS) data were processed with Proteome Discoverer 2.4 (Thermo Fisher Scientific). Tandem mass spectra were searched in the Human Uniprot database (88473 sequences, v 09.2015) with the enzyme digestion method set as Trypsin/P and the number of missing bits set to 2. The mass tolerance for precursor ions was set as 20 ppm and 5 ppm for the first search and main search, respectively, with a mass tolerance for fragment ions of 0.02 Da. The peptide false-discovery rate (FDR) was set at 1%, and only proteins with at least two unique peptides per protein per sample were considered as positively identified.
Bioinformatics analyses
Proteins were considered to be differentially abundant between samples with a fold change value ≥1.5 or ≤0.67 and P<0.05. Identified differentially abundant proteins were subjected to gene ontology (GO) annotation analyses, in which two-tailed Fisher’s exact tests were used to test the enrichment of these proteins. GO annotations were divided into three categories: Molecular Function (MF), Biological Process (BP), and Cellular Components (CC). Further, the enrichment of the differentially abundant proteins in specific Kyoto Encyclopedia of Genes and Genomes (KEGG) pathways was assessed through two-tailed Fisher’s exact tests, with a corrected P value of <0.05 as the significance threshold. Protein-protein interaction (PPI) networks incorporating the differentially abundant proteins were constructed using the STRING database, and high confidence relationships (score >0.7) were extracted.
Statistical analysis
Data are presented as mean ± standard deviation (SD). Student’s t-tests were used for comparisons between groups, and the significance threshold was P<0.05.
Results
Patient characteristics
The BD group included 5 males and 3 females with an average age of 4.85±4.07 years (range, 0.8–11 years). The HC group included 3 males and 5 females with an average age of 4.80±2.70 years (range, 1.6–8 years). There were no significant differences in age or sex between the two groups (P>0.05). Causes of BD among the participating patients included severe traumatic brain injury (n=3), encephalitis (n=2), and brain tumor, drowning, and severe pneumonia (n=1 each). Six of the patients had been transferred from other hospitals and entered a deep coma for 3–39 days (median: 11 days) after BD diagnosis. More clinical details of the patients can be seen in Table 1.
Table 1
Patient no. | Age (years) | Sex | Time from coma to BD diagnosis (days) | Cause of BD |
---|---|---|---|---|
1 | 8 | Male | 39 | Encephalitis |
2 | 2 | Male | 8 | Brain tumor |
3 | 0.8 | Male | 5 | Drowning |
4 | 1 | Male | 3 | Severe traumatic brain injury |
5 | 1 | Female | 14 | Encephalitis |
6 | 7 | Male | 10 | Severe pneumonia |
7 | 8 | Female | 12 | Severe traumatic brain injury |
8 | 11 | Female | 15 | Severe traumatic brain injury |
BD, brain death.
Differential protein identification and quantitative analysis
A total of 490 serum proteins were identified and quantified by proteomic analysis (Figure 1), 67 of which exhibited clear differential expression between the BD and HC groups. These 67 proteins were subsequently screened: 29 were upregulated and 38 were downregulated (Table 2). The 5 most significantly upregulated proteins in the serum of patients with BD were lipopolysaccharide-binding protein (LBP), α1-acid glycoprotein (α1-AGP), α1-antichymotrypsin (α1-AAT), leucine-rich α1-glycoprotein (LRG1), and lactate dehydrogenase B heavy chain (LDHB), which were upregulated by fold change values of 3.55, 3.18, 3.16, 3.00, and 2.92, respectively (P<0.05). The 5 most significantly downregulated proteins in the serum of patients with BD were actin-binding protein 2 (transgelin-2), platelet basic protein (PBP), tropomyosin α4 chain (TPM4), tropomyosin α3 chain (TPM3), and peptidase inhibitor 16 (PI16), which had fold change values of 0.27, 0.29, 0.30, 0.33, and 0.35, respectively (P<0.05).
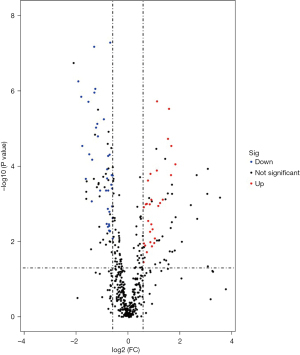
Table 2
Accession no. | Protein | Gene symbol | Up-/down-regulation | Fold change | P value |
---|---|---|---|---|---|
P37802 | Transgelin-2 | TAGLN2 | Down | 0.266 | 0.000 |
P02775 | Platelet basic protein | PPBP | Down | 0.287 | 0.000 |
P67936 | Tropomyosin alpha-4 chain | TPM4 | Down | 0.295 | 0.000 |
P06753 | Tropomyosin alpha-3 chain | TPM3 | Down | 0.325 | 0.000 |
Q6UXB8 | Peptidase inhibitor 16 | PI16 | Down | 0.348 | 0.000 |
P02647 | Apolipoprotein A-I | APOA1 | Down | 0.356 | 0.000 |
P02652 | Apolipoprotein A-II | APOA2 | Down | 0.381 | 0.001 |
Q9Y490 | Talin-1 | TLN1 | Down | 0.385 | 0.000 |
Q9NQ79 | Cartilage acidic protein-1 | CRTAC1 | Down | 0.406 | 0.000 |
P05452 | Tetranectin | CLEC3B | Down | 0.408 | 0.000 |
P06396 | Gelsolin | GSN | Down | 0.418 | 0.000 |
Q13790 | Apolipoprotein F | APOF | Down | 0.426 | 0.000 |
P07996 | Thrombospondin-1 | THBS1 | Down | 0.442 | 0.000 |
P27169 | Paraoxonase/arylesterase-1 | PON1 | Down | 0.449 | 0.000 |
P35443 | Thrombospondin-4 | THBS4 | Down | 0.475 | 0.000 |
P11597 | Cholesteryl ester transfer protein | CETP | Down | 0.526 | 0.000 |
P40197 | Platelet glycoprotein V | GP5 | Down | 0.558 | 0.000 |
P02649 | Apolipoprotein E | APOE | Down | 0.566 | 0.003 |
Q9UHG3 | Prenylcysteine oxidase 1 | PCYOX1 | Down | 0.573 | 0.000 |
P80108 | Phosphatidylinositol-glycan-specific phospholipase D | GPLD1 | Down | 0.573 | 0.000 |
P49908 | Selenoprotein P | SELENOP | Down | 0.584 | 0.001 |
Q9UGM5 | Fetuin-B | FETUB | Down | 0.586 | 0.004 |
Q86YW5 | Trem-like transcript 1 protein | TREML1 | Down | 0.589 | 0.000 |
P03952 | Plasma kallikrein | KLKB1 | Down | 0.591 | 0.000 |
Q04756 | Hepatocyte growth factor activator | HGFAC | Down | 0.603 | 0.003 |
Q15063 | Periostin | POSTN | Down | 0.604 | 0.005 |
P05154 | Plasma serine protease inhibitor | SERPINA5 | Down | 0.606 | 0.004 |
P55058 | Phospholipid transfer protein | PLTP | Down | 0.609 | 0.002 |
P51884 | Lumican | LUM | Down | 0.613 | 0.000 |
P06276 | Cholinesterase | BCHE | Down | 0.619 | 0.005 |
P08294 | Extracellular superoxide dismutase | SOD3 | Down | 0.623 | 0.000 |
P05067 | Amyloid-beta precursor protein | APP | Down | 0.641 | 0.000 |
P29622 | Kallistatin | SERPINA4 | Down | 0.648 | 0.000 |
P03951 | Coagulation factor XI | F11 | Down | 0.655 | 0.000 |
P55290 | Cadherin-13 | CDH13 | Down | 0.655 | 0.001 |
P07359 | Platelet glycoprotein Ib alpha chain | GP1BA | Down | 0.664 | 0.002 |
P14151 | L-selectin | SELL | Down | 0.666 | 0.000 |
P05090 | Apolipoprotein D | APOD | Down | 0.666 | 0.008 |
P0C0L4 | Complement C4-A | C4A | Up | 1.520 | 0.035 |
P05155 | Plasma protease C1 inhibitor | SERPING1 | Up | 1.529 | 0.001 |
Q92954 | Proteoglycan 4 | PRG4 | Up | 1.533 | 0.011 |
P00742 | Coagulation factor X | F10 | Up | 1.584 | 0.012 |
Q9UK55 | Protein Z-dependent protease inhibitor | SERPI | Up | 1.609 | 0.001 |
P18206 | Vinculin | VCL | Up | 1.655 | 0.001 |
P20742 | Pregnancy zone protein | PZP | Up | 1.657 | 0.019 |
Q14624 | Inter-alpha-trypsin inhibitor heavy chain H4 | ITIH4 | Up | 1.710 | 0.000 |
P48637 | Glutathione synthetase | GSS | Up | 1.718 | 0.003 |
P19652 | Alpha-1-acid glycoprotein 2 | ORM2 | Up | 1.791 | 0.001 |
P09871 | Complement C1s | C1S | Up | 1.806 | 0.005 |
P09960 | Leukotriene A-4 hydrolase | LTA4H | Up | 1.821 | 0.010 |
Q15485 | Ficolin-2 | FCN2 | Up | 1.823 | 0.000 |
P33908 | Mannosyl-oligosaccharide 1,2-alpha-mannosidase IA | MAN1A1 | Up | 1.865 | 0.001 |
P11021 | Endoplasmic reticulum chaperone BiP | HSPA5 | Up | 1.866 | 0.003 |
P06732 | Creatine kinase M-type | CKM | Up | 1.898 | 0.013 |
P00736 | Complement C1r | C1R | Up | 1.932 | 0.005 |
P01833 | Polymeric immunoglobulin receptor | PIGR | Up | 2.018 | 0.011 |
Q02985 | Complement factor H-related protein 3 | CFHR3 | Up | 2.051 | 0.008 |
Q06033 | Inter-alpha-trypsin inhibitor heavy chain H3 | ITIH3 | Up | 2.165 | 0.000 |
P02748 | Complement component C9 | C9 | Up | 2.176 | 0.000 |
P01009 | Alpha-1-antitrypsin | SERPINA1 | Up | 2.248 | 0.001 |
P02743 | Serum amyloid P-component | APCS | Up | 2.356 | 0.001 |
P14618 | Pyruvate kinase | PKM | Up | 2.538 | 0.001 |
P07195 | L-lactate dehydrogenase B chain | LDHB | Up | 2.917 | 0.000 |
P02750 | Leucine-rich alpha-1-glycoprotein | LRG1 | Up | 3.002 | 0.000 |
P01011 | Alpha-1-antichymotrypsin | SERPINA3 | Up | 3.160 | 0.000 |
P02763 | Alpha-1-acid glycoprotein 1 | ORM1 | Up | 3.184 | 0.000 |
P18428 | Lipopolysaccharide binding protein | LBP | Up | 3.554 | 0.000 |
Functional enrichment analyses
Next, GO functional annotation analyses were conducted for all proteins identified in the quantitative analysis, and GO enrichment analyses of differentially abundant proteins between the BD and HC samples were performed using Fisher’s exact test. The differentially abundant proteins were then classified into three categories which included BP, CC, and MF GO categories (Figure 2): enriched BP terms included “cellular processes”, “biological regulation”, “metabolic processes”, and “responses to stimulus” (P>0.05); enriched CC terms were primarily associated with cellular anatomical entities, protein-containing complexes, and other organism parts (P<0.05); and enriched MF terms included “binding”, “molecular function regulators”, “catalytic”, “structural molecule”, and “molecular transducer” activities (P<0.05).
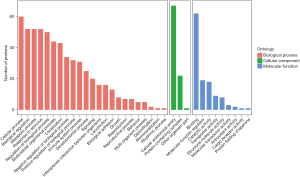
To further establish the functional roles of the differentially abundant proteins, a KEGG pathway enrichment analysis was performed. The analysis revealed that the proteins were enriched in 78 pathways, the top 20 of which are represented by bubble charts in Figure 3. No chemotaxis-related functional enhancement was evident when the two groups were compared; however, several of the differentially abundant proteins are related to the complement and coagulation cascades, with 11 of them being involved in these pathways. Also, 7 of the differentially abundant proteins are involved in the cholesterol metabolism pathway. Associations of LBP, LDHB, and PPB with the Toll-like receptor, cancer central carbon metabolism, and chemokine signaling pathways, respectively, were also found.
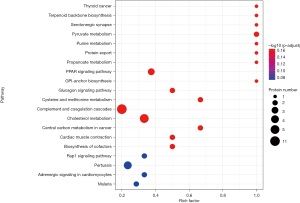
Hierarchical clustering analyses revealed clear separation between the 67 proteins, thus further supporting the rationality and accuracy of this screening approach for differentially abundant proteins as a method for distinguishing serum samples of patients with BD from those of healthy individuals (Figure 4).

PPI network analysis
Finally, interactions among the differentially abundant proteins were analyzed with the STRING database, and putative interaction relationships with a high confidence threshold (>0.7) were extracted to construct a PPI network (Figure 5). A network of 67 nodes (proteins) and 262 edges (interactions) was produced, with 132 edges exhibiting a combined score of >0.7. Interestingly, a predicted interaction between pyruvate kinase muscle isozyme and LDHB was evident, with a significant interaction score of 0.958.
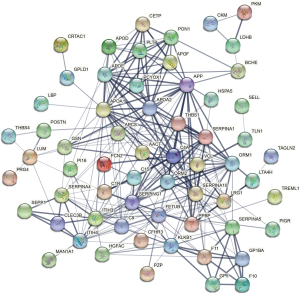
Discussion
Blood can offer invaluable insight into systemic health and the mechanisms governing a disease state. In the present study, proteins associated with the occurrence of BD were identified through comparison of serum samples from children diagnosed with BD and those from HCs. In total, 490 serum proteins were detected in the samples, of which 67 were differentially abundant between the two groups. Of these 67 proteins, 11 were involved in the complement and coagulation cascade pathways and 7 were involved in the cholesterol metabolism pathway. In total, 29 proteins were upregulated in the serum of the BD group, with LBP, α1-AGP, α1-ACT, LRG1, and LDHB being those most significantly upregulated. There were 38 downregulated proteins in the serum of patients with BD, with transgelin-2, PBP, TPM4, TPM3, and PI16 being those most significantly downregulated.
Differential expression dynamics can offer insight into physiological changes related to shifts in cellular activity (12). Two previous studies of liver and kidney tissue samples from a rabbit model of BD revealed that protein expression changes may be a sensitive predictor of donor organ quality in patients with BD (13,14). Further, a study of the rostral ventrolateral medulla of rats were killed that revealed that antioxidant proteins were significantly upregulated when compared to cerebral cortex and were associated with protection against BD-related damage (15). The changes in protein abundance observed in the present study may therefore be linked to the molecular processes governing BD pathogenesis.
LBP is an acute-phase glycoprotein produced by hepatocytes that exhibits a high affinity for the lipid A component of lipopolysaccharide (LPS) produced by Gram-negative bacteria (16). LBP and its ligand CD14 are upstream regulators of LPS-induced inflammatory activity, and can induce systemic inflammation septicemia, multiple organ dysfunction syndrome, and acute lung injury (17-19). Disrupting interactions between LBP and CD14 may suppress LPS-induced inflammatory activity (20). In this study, LBP was found to be enriched in the Toll-like receptor pathway, which is consistent with a role for this protein as a regulator of inflammatory cascades associated with BD.
Alpha 1-AGP, which is primarily produced by liver macrophages and granulocytes, is one of the most important glycoproteins in serum. In addition to regulating drug pharmacokinetics and pharmacodynamics, this protein also binds to and transports a variety of inflammation-related ligands. It can modulate diverse inflammatory processes, including pathogen binding and leukocyte-mediated pathogen killing (21). Significant rises in serum α1-AGP concentrations increases reflect acute inflammation, which can be observed during acute-phase reactions associated with myocardial infarction, septicemia, inflammatory lung diseases, and other conditions (22). Previous studies demonstrated utilizing α1-AGP also serve as an index for monitoring chronic inflammatory activity (23). A protein metabolomics study of 106 candidate biomarkers identified α1-AGP as the strongest multivariate predictor for the risk of all-cause death within 5 years in adults (24).
α1-ACT is an acute-phase protein and serine protease inhibitor produced mainly by the liver that inhibits chymotrypsin-like enzymes, particularly neutrophil cathepsin G (25). As a proteolytic enzyme, cathepsin G reportedly may lead to the degradation of extracellular matrix (26). Furthermore, α1-ACT might play a role in the pathophysiology of BD through regulating the activity of cathepsin G.
LRG1 is a novel proangiogenic factor that plays a role in inflammation and abnormal angiogenesis by upregulating transforming growth factor β (TGF-β) signaling (27). LRG1 and TGF-β have been linked to the pathogeneses of heart failure, myocardial infarction, diabetic nephropathy, respiratory infections, and inflammatory bowel disease, all of which involve enhanced endothelial cell migration and the associated induction of angiogenesis as mechanisms of disease progression (28-31). LRG1 overexpression has often been linked to poor prognosis, suggesting that it may play a role in BD.
LDHB is an important glycolytic enzyme that facilitates anaerobic metabolism and the conversion of lactate into glucose. LDHB and PKM have been reported to be promising CSF biomarkers in patients with Alzheimer’s disease (AD) (32), with both being upregulated in CSF samples from patients with the disease, suggesting that they may be indicative of a reverse Warburg effect that contributes to disease progression (33). After LDHB, PKM was the next most upregulated protein in the serum of BD patients in this study, and a putative interaction between PKM and LDHB was noted in the PPI network analysis, which suggests a potential role for the reverse Warburg effect in the pathogenesis of BD.
Transgelin-2 is a small 22-kDa actin-binding protein that stabilizes synapse formation between T cells and antigen-presenting cells, regulates actin dynamics, and is involved in bacterial phagocytosis by macrophages (34). Reduced transgelin-2 levels in B cells can lead to T cell attenuation (35), and may result in the induction of pro-inflammatory and pro-chondrogenic changes in vascular smooth muscle cells (36).
PBP is a specific protein found in megakaryocytes and platelets that is involved in both signal transduction and antibacterial activity, playing key roles in innate immune responses (37). The biological role of PBP depends on its derivatives (CTA-III, β-TG, and NAP-2), which serve as mediators of inflammation and wound healing, and exhibit antibacterial and antifungal activity (38,39). In one report, the post-BD infection rate was high (40). PBP downregulation in patients with BD may result in weakening of the antibacterial immune response at the cellular level, increasing the odds of subsequent infection.
TPM is a thin myofilament-related protein, the downregulation of which may result in cytoskeletal reorganization, altered cellular morphology, and changes in the epithelial-mesenchymal transition process related to cancer and fibrotic conditions (41).
PI16 belongs to a family of proteins which are abundant in cysteine secretions and are be highly upregulated in cardiac disease and prostate cancer. Earlier work suggests that PI16 plays important roles in autoimmunity by influencing regulatory T cells to shape pro-inflammatory responses at inflamed tissue sites (42,43). To date, there have been few studies of PI16, and its mechanistic importance in physiological and pathological contexts remains to be defined.
In this study, we analyzed the serum proteome from patients with BD and age- and sex-matched HCs. Through our analysis, we identified 67 differentially abundant proteins between the two groups. Most, if not all, of these proteins have been reported in human serum previously. Many of the patients included in the BD group in this study had been hospitalized for extended periods at other institutions not qualified to diagnose BD before being transferred to our hospital. Given the inherent difficulties in establishing a diagnosis of BD, samples were collected from the patients in this study at different time points after BD had been diagnosed. Such temporal differences may have affected the final protein expression outcomes. Significant brain injury of any aetiology will cause a systemic response, creating a proinflammatory environment prior to the occurrence of BD itself (44), which augment the inflammation cascade reaction and cause serious damage to respiration and circulation. Respiration, circulation, and brain functions are interdependent, with any system shutting down and the rest inevitably shutting down within minutes. Therefore, it is very important to block the development of systemic inflammatory response syndrome (SIRS). Systemic inflammatory response in BD is triggered mainly by BD itself rather than by other injuries that develop during critical illness (45). Inflammation secondary to BD can adversely affect the graft quality after donation of their organs. We retrospectively analyzed 10 significantly differentially abundant proteins in the collected serum samples and found them to be related to inflammation. This finding suggests that BD may warrant anti-inflammatory treatment, although a clearer understanding of the associated mechanisms and pathways is essential to prevent excessive BD-related organ damage. PPI network analyses further revealed that the differentially abundant proteins formed a network of 67 nodes and 262 edges, with 132 edges exhibiting a combined score of >0.7. However, inflammation-related proteins were not enriched in KEGG pathway analyses, which suggests that more work is required to understand the complexity and pathophysiology of BD.
In conclusion, this proteomics analysis of serum samples from patients with BD and HCs revealed clear BD-related differences in serum protein profiles. Previous research has indicated that many differentially abundant proteins can influence the pathogenesis of diverse disease states, and significant differential abundance was observed for a variety of inflammation-associated proteins in the present study. Therefore, our findings may offer new insights into the occurrence of BD, and have the potential to serve as a foundation for establishing reliable biomarkers for this devastating condition. Further research into the molecular mechanisms linking these putative biomarkers to BD pathogenesis is warranted to aid better understanding of how to detect and potentially prevent this condition or associated organ damage.
Acknowledgments
The authors would like to thank all the reviewers who participated in the review of this article, and Dr. Dev Sooranna of Imperial College London and MJEditor (www.mjeditor.com) for their linguistic assistance during the preparation of this manuscript.
Funding: This study was supported by Nanning Qingxiu District Science and Technology Bureau (No. 2020049).
Footnote
Reporting Checklist: The authors have completed the MDAR reporting checklist. Available at https://tp.amegroups.com/article/view/10.21037/tp-21-559/rc
Data Sharing Statement: Available at https://tp.amegroups.com/article/view/10.21037/tp-21-559/dss
Conflicts of Interest: All authors have completed the ICMJE uniform disclosure form (available at https://tp.amegroups.com/article/view/10.21037/tp-21-559/coif). The authors have no conflicts of interest to declare.
Ethical Statement: The authors are accountable for all aspects of the work in ensuring that questions related to the accuracy or integrity of any part of the work are appropriately investigated and resolved. The study was conducted according to the guidelines of the Declaration of Helsinki (as revised in 2013), and was approved by the Medical Ethics Committee of the First Affiliated Hospital of Guangxi Medical University [project identification code: 2020 (KY-E-156)]. Signed written informed consent was obtained from the parents or guardians of each participating child before study enrollment.
Open Access Statement: This is an Open Access article distributed in accordance with the Creative Commons Attribution-NonCommercial-NoDerivs 4.0 International License (CC BY-NC-ND 4.0), which permits the non-commercial replication and distribution of the article with the strict proviso that no changes or edits are made and the original work is properly cited (including links to both the formal publication through the relevant DOI and the license). See: https://creativecommons.org/licenses/by-nc-nd/4.0/.
References
- Xue W, Tian P, Xiang H, et al. Outcomes for primary kidney transplantation from donation after Citizens' death in China: a single center experience of 367 cases. BMC Health Serv Res 2017;17:250. [Crossref] [PubMed]
- Huang J, Millis JM, Mao Y, et al. Voluntary organ donation system adapted to Chinese cultural values and social reality. Liver Transpl 2015;21:419-22. [Crossref] [PubMed]
- Avlonitis VS, Wigfield CH, Kirby JA, et al. The hemodynamic mechanisms of lung injury and systemic inflammatory response following brain death in the transplant donor. Am J Transplant 2005;5:684-93. [Crossref] [PubMed]
- Pelletier SJ, Guidinger MK, Merion RM, et al. Recovery and utilization of deceased donor kidneys from small pediatric donors. Am J Transplant 2006;6:1646-52. [Crossref] [PubMed]
- van der Hoeven JA, Molema G, Ter Horst GJ, et al. Relationship between duration of brain death and hemodynamic (in)stability on progressive dysfunction and increased immunologic activation of donor kidneys. Kidney Int 2003;64:1874-82. [Crossref] [PubMed]
- Kirschen MP, Francoeur C, Murphy M, et al. Epidemiology of Brain Death in Pediatric Intensive Care Units in the United States. JAMA Pediatr 2019;173:469-76. [Crossref] [PubMed]
- Reiber H. Dynamics of brain-derived proteins in cerebrospinal fluid. Clin Chim Acta 2001;310:173-86. [Crossref] [PubMed]
- Pan S, Chen R, Crispin DA, et al. Protein alterations associated with pancreatic cancer and chronic pancreatitis found in human plasma using global quantitative proteomics profiling. J Proteome Res 2011;10:2359-76. [Crossref] [PubMed]
- Zhang X, Shi S, Zhang B, et al. Circulating biomarkers for early diagnosis of pancreatic cancer: facts and hopes. Am J Cancer Res 2018;8:332-53. [PubMed]
- Gao W, Lu C, Kochanek PM, et al. Serum amyloid A is increased in children with abusive head trauma: a gel-based proteomic analysis. Pediatr Res 2014;76:280-6. [Crossref] [PubMed]
- Qian S, Su Y. Criteria and practical guidance for determination of brain death in children. Zhonghua Er Ke Za Zhi 2019;57:331-5. [PubMed]
- Millar RP, Newton CL. The year in G protein-coupled receptor research. Mol Endocrinol 2010;24:261-74. [Crossref] [PubMed]
- Du B, Li L, Zhong Z, et al. Brain death induces the alteration of liver protein expression profiles in rabbits. Int J Mol Med 2014;34:578-84. [Crossref] [PubMed]
- Li L, Li N, He C, et al. Proteomic analysis of differentially expressed proteins in kidneys of brain dead rabbits. Mol Med Rep 2017;16:215-23. [Crossref] [PubMed]
- Chou JL, Wu CH, Tsai CY, et al. Proteomic investigation of a neural substrate intimately related to brain death. Proteomics 2011;11:239-48. [Crossref] [PubMed]
- Schumann RR, Leong SR, Flaggs GW, et al. Structure and function of lipopolysaccharide binding protein. Science 1990;249:1429-31. [Crossref] [PubMed]
- Hotchkiss RS, Moldawer LL, Opal SM, et al. Sepsis and septic shock. Nat Rev Dis Primers 2016;2:16045. [Crossref] [PubMed]
- Chimenti L, Morales-Quinteros L, Puig F, et al. Comparison of direct and indirect models of early induced acute lung injury. Intensive Care Med Exp 2020;8:62. [Crossref] [PubMed]
- Jabandziev P, Smerek M, Michalek J, et al. Multiple gene-to-gene interactions in children with sepsis: a combination of five gene variants predicts outcome of life-threatening sepsis. Crit Care 2014;18:R1. [Crossref] [PubMed]
- Fang L, Xu Z, Wang GS, et al. Directed evolution of an LBP/CD14 inhibitory peptide and its anti-endotoxin activity. PLoS One 2014;9:e101406. [Crossref] [PubMed]
- Ceciliani F, Lecchi C. The Immune Functions of α1 Acid Glycoprotein. Curr Protein Pept Sci 2019;20:505-24. [Crossref] [PubMed]
- Gornik O, Lauc G. Glycosylation of serum proteins in inflammatory diseases. Dis Markers 2008;25:267-78. [Crossref] [PubMed]
- Suchdev PS, Williams AM, Mei Z, et al. Assessment of iron status in settings of inflammation: challenges and potential approaches. Am J Clin Nutr 2017;106:1626S-33S. [Crossref] [PubMed]
- Fischer K, Kettunen J, Würtz P, et al. Biomarker profiling by nuclear magnetic resonance spectroscopy for the prediction of all-cause mortality: an observational study of 17,345 persons. PLoS Med 2014;11:e1001606. [Crossref] [PubMed]
- Wågsäter D, Johansson D, Fontaine V, et al. Serine protease inhibitor A3 in atherosclerosis and aneurysm disease. Int J Mol Med 2012;30:288-94. [Crossref] [PubMed]
- Lu P, Takai K, Weaver VM, et al. Extracellular matrix degradation and remodeling in development and disease. Cold Spring Harb Perspect Biol 2011;3:a005058. [Crossref] [PubMed]
- Zhang A, Fang H, Chen J, et al. Role of VEGF-A and LRG1 in Abnormal Angiogenesis Associated With Diabetic Nephropathy. Front Physiol 2020;11:1064. [Crossref] [PubMed]
- Hong Q, Zhang L, Fu J, et al. LRG1 Promotes Diabetic Kidney Disease Progression by Enhancing TGF-β-Induced Angiogenesis. J Am Soc Nephrol. 2019;30:546-62. [Crossref] [PubMed]
- Song W, Wang X. The role of TGFβ1 and LRG1 in cardiac remodelling and heart failure. Biophys Rev 2015;7:91-104. [Crossref] [PubMed]
- Honda H, Fujimoto M, Miyamoto S, et al. Sputum Leucine-Rich Alpha-2 Glycoprotein as a Marker of Airway Inflammation in Asthma. PLoS One 2016;11:e0162672. [Crossref] [PubMed]
- Wang Y, Xu J, Zhang X, et al. TNF-α-induced LRG1 promotes angiogenesis and mesenchymal stem cell migration in the subchondral bone during osteoarthritis. Cell Death Dis 2017;8:e2715. [Crossref] [PubMed]
- Johnson ECB, Dammer EB, Duong DM, et al. Large-scale proteomic analysis of Alzheimer's disease brain and cerebrospinal fluid reveals early changes in energy metabolism associated with microglia and astrocyte activation. Nat Med 2020;26:769-80. [Crossref] [PubMed]
- Bonuccelli G, Whitaker-Menezes D, Castello-Cros R, et al. The reverse Warburg effect: glycolysis inhibitors prevent the tumor promoting effects of caveolin-1 deficient cancer associated fibroblasts. Cell Cycle 2010;9:1960-71. [Crossref] [PubMed]
- Jo S, Kim HR, Mun Y, et al. Transgelin-2 in immunity: Its implication in cell therapy. J Leukoc Biol 2018;104:903-10. [Crossref] [PubMed]
- Na BR, Kwon MS, Chae MW, et al. Transgelin-2 in B-Cells Controls T-Cell Activation by Stabilizing T Cell - B Cell Conjugates. PLoS One 2016;11:e0156429. [Crossref] [PubMed]
- Shen J, Yang M, Jiang H, et al. Arterial injury promotes medial chondrogenesis in Sm22 knockout mice. Cardiovasc Res 2011;90:28-37. [Crossref] [PubMed]
- Singh A, Bisht P, Bhattacharya S, et al. Role of Platelet Cytokines in Dengue Virus Infection. Front Cell Infect Microbiol 2020;10:561366. [Crossref] [PubMed]
- Tang Z, Yu M, Miller F, et al. Increased invasion through basement membrane by CXCL7-transfected breast cells. Am J Surg 2008;196:690-6. [Crossref] [PubMed]
- Cheng Y, Ma XL, Wei YQ, et al. Potential roles and targeted therapy of the CXCLs/CXCR2 axis in cancer and inflammatory diseases. Biochim Biophys Acta Rev Cancer 2019;1871:289-312. [Crossref] [PubMed]
- Wang HY, Liang SF. Maintenance and Treatment of Organs from Brain-Dead Donors with Infection. Practical Clinical Medicine 2019;20:24-6.
- Gervasi M, Bianchi-Smiraglia A, Cummings M, et al. JunB contributes to Id2 repression and the epithelial-mesenchymal transition in response to transforming growth factor-β. J Cell Biol 2012;196:589-603. [Crossref] [PubMed]
- Grose RH, Millard DJ, Mavrangelos C, et al. Comparison of blood and synovial fluid th17 and novel peptidase inhibitor 16 Treg cell subsets in juvenile idiopathic arthritis. J Rheumatol 2012;39:2021-31. [Crossref] [PubMed]
- Nicholson IC, Mavrangelos C, Bird DR, et al. PI16 is expressed by a subset of human memory Treg with enhanced migration to CCL17 and CCL20. Cell Immunol 2012;275:12-8. [Crossref] [PubMed]
- Watts RP, Thom O, Fraser JF. Inflammatory signalling associated with brain dead organ donation: from brain injury to brain stem death and posttransplant ischaemia reperfusion injury. J Transplant 2013;2013:521369. [Crossref] [PubMed]
- Schwarz P, Custódio G, Rheinheimer J, et al. Brain Death-Induced Inflammatory Activity is Similar to Sepsis-Induced Cytokine Release. Cell Transplant 2018;27:1417-24. [Crossref] [PubMed]